The internet has been transformative for AI development, providing the vast quantities of data that power today’s most advanced systems.
From language models to computer vision, many of modern AI’s breakthroughs would be impossible without access to this enormous pool of digital information.
However, more data doesn’t always mean better AI. While the internet offers a seemingly endless resource of topical and relevant data, abundance masks a critical challenge: distinguishing valuable information from noise.
Raw internet data is a messy mix of facts, opinions, outdated information, and sometimes harmful content – all of which can be detrimental when entering AI systems.
Let’s review some key challenges of training AI systems on general but plentiful data sources and why domain-specific data is key for building dependable systems.
The Problems of Generalist Data
When training AI models, especially large language models (LLMs), the internet provides a colossal volume of data on virtually every topic relevant to humanity.
However, training AI models with internet content without careful filtering or supplementation from other sources can cause AI to inherit inaccuracies, outdated information, and harmful biases.
This challenge isn’t unique to LLMs. Other AI systems, like computer vision or recommendation models that also depend on internet-sourced, general, or open-source datasets, face similar limitations.
Let’s review two key challenges of training AI systems on general but plentiful data sources:
1. Quality and Validation Challenges
The first major challenge with using internet-sourced data is the lack of quality control. While the internet provides access to a vast range of information, it also includes outdated, biased, misleading, or even offensive content.
With no catch-all mechanisms for verifying accuracy or filtering out unreliable sources, AI systems can easily absorb and reproduce inaccuracies, leading to flawed outputs.
The LAION (Large-scale AI Open Network) datasets, containing over 5.8 billion image-text pairs, perfectly illustrate this challenge. While this massive collection powers many modern AI image generators and vision systems, it’s faced criticism for insufficient content validation.
David Thiel, the chief technologist at the Stanford Internet Observatory, investigated LAION and its shortcomings issues, describing:
“Taking an entire internet-wide scrape and making that dataset to train models is something that should have been confined to a research operation, if anything, and is not something that should have been open-sourced without a lot more rigorous attention.”
When we rely on open-source datasets like LAION, we risk creating models that pick up biases or harmful content. This can lead to outputs that aren’t just incorrect but potentially dangerous.
2. Outdated and Biased Data
In addition to quality concerns, generalist datasets like Tiny Images and LAION also suffer from representation gaps and outdated data.
The MIT Tiny Images dataset, widely used in computer vision tasks, was found to contain offensive labels and imagery that reflected outdated societal views and biases.
Worse still, AI models trained on such datasets lack the flexibility of human cognition to update their understanding based on evolving values and standards.
A 2020 investigation by The Register revealed that the Tiny Images dataset contained numerous offensive labels, including obscene, racist, and sexist terms.
Antonio Torralba from MIT, the lab responsible for the dataset, responded to the criticism, “It is clear that we should have manually screened them.”
After this discovery, MIT issued a statement announcing that the dataset had been removed from public access.
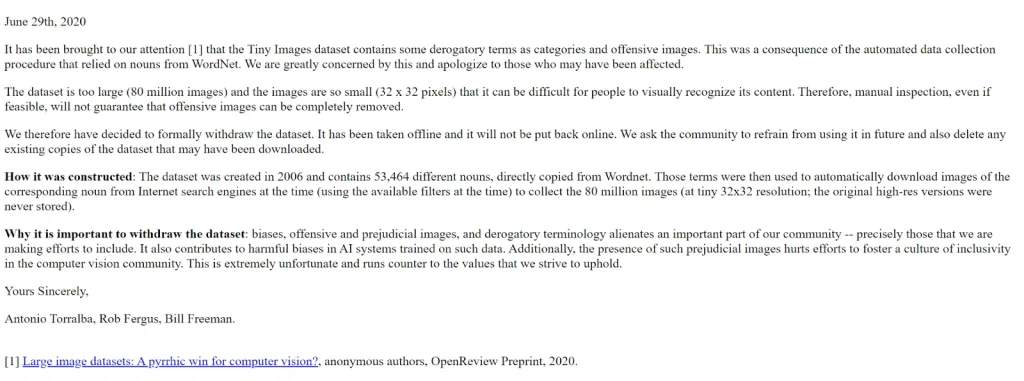
Above: MIT’s statement about Tiny Images. Source.
AI in Medicine: A Case Study
Stanford researchers recently tested popular AI models like ChatGPT and Google’s Bard on medical questions, uncovering consistent patterns of dangerous misinformation.
The AIs confidently promoted debunked racial theories about kidney function, cited discredited 19th-century ideas about lung capacity, and recommended different treatment standards based purely on race.
The implications are serious. These models routinely:
- Calculate medication dosages using outdated racial formulae
- Underestimate pain levels for minority patients
- Make unfounded assumptions about disease susceptibility
- Recommend lower standards of care for certain demographic groups
Stanford University’s Dr Roxana Daneshjou told Fortune, “There are very real-world consequences to getting this wrong that can impact health disparities,” adding, “We are trying to have those tropes removed from medicine, so the regurgitation of that is deeply concerning.”
Developing AI with Deep Domain Expertise
General datasets offer a broad range of information but often lack the depth and precision needed for specialised AI applications. In fields like healthcare, finance, and law, AI requires data that is both accurate and specifically aligned with the unique demands of each industry.
So, how do we source and build the right data to ensure AI performs effectively in these areas?
1. Source Reliable, Specialised Data
Building high-quality, industry-specific data goes beyond gathering information from the web. It means sourcing reliable data, refining it, and working closely with experts and trusted institutions to create a solid foundation tailored to your industry.
The goal is to equip AI with detailed, trustworthy information that leads to clear, actionable insights instead of vague, general conclusions.
2. Collect Data From Real-World Environments
Often, developing domain-specific AI requires collecting data directly from real-world settings, which can be challenging when data isn’t readily available online or is limited to certain institutions or locations.
For example, one of Aya Data’s agriculture projects in Ghana involved gathering over 5,000 images of maize plants in rural areas to train an AI system for disease detection. This data had to be collected on-site under real-world conditions.
3. Consider Industry-Specific Constraints
Different industries face unique challenges when collecting the specialised data needed for domain-specific AI:
- Healthcare: Strict privacy regulations make it difficult to access patient data, creating barriers to obtaining the detailed information AI models need for accurate outcomes. It’s possible to collect ethical health data by partnering with established medical institutions, as demonstrated by Aya Data’s collaboration with the University of Ghana Medical Centre.
- Finance: Tight regulations around data sharing and confidentiality make financial institutions reluctant to provide access to critical datasets, complicating efforts to train effective AI models.
- Legal: Confidentiality restrictions and legal protections limit access to comprehensive legal datasets, making it challenging to gather the data required for AI systems to handle complex legal processes.
RAG: Making Domain Knowledge Work
Today’s AI models are powerful but fundamentally limited: They can’t learn anything new after training. Once deployed, their knowledge is frozen in time – a serious problem when you need current, accurate information.
Retrieval Augmented Generation (RAG) enables AI systems to access domain-specific knowledge bases in real-time.
A RAG-enabled system can access and incorporate relevant information from medical research, technical documentation, or company databases while processing queries.
How RAG Works
RAG enhances AI’s ability to deliver precise answers by combining pre-trained knowledge with up-to-date data retrieval. Here’s how it works:
- Interpreting user intent: When a user submits a query, the system first determines the context and intent behind the question, ensuring a focused response.
- Retrieving relevant information with vector databases: RAG then taps into vector databases, a specialised type of database that enables deeper search functionality. Unlike traditional databases that store data as plain text, vector databases convert information into “embeddings” – numerical representations that capture the meaning and context of words and phrases. This allows the system to identify and retrieve data based on conceptual relevance.
- Sourcing specialised knowledge: With vector-based retrieval, RAG pulls relevant information from trusted sources like medical research, technical manuals, or internal databases, going beyond pre-trained data to offer precision in specialised domains.
- Integrating real-time data with pre-trained knowledge: Finally, RAG combines retrieved information with the system’s pre-existing knowledge, enabling responses that are both current and specific to the query.
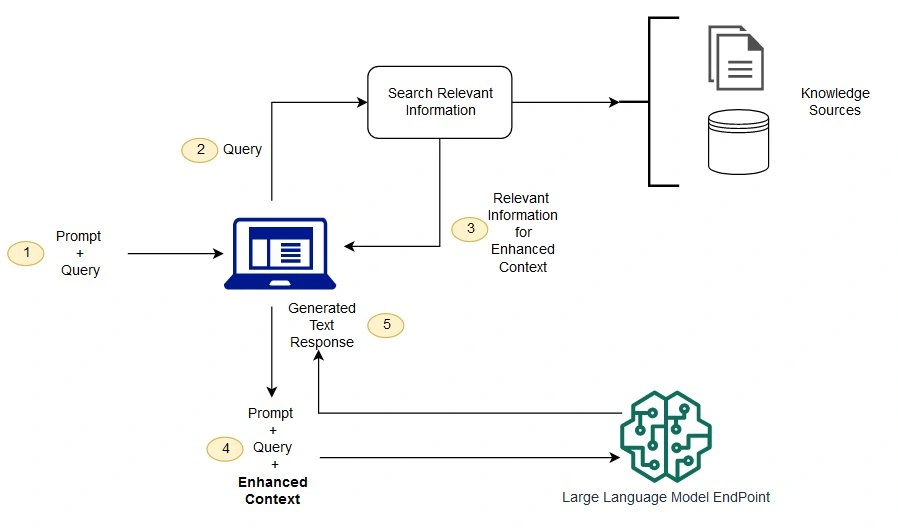
The Business Case for RAG
When AI gets it wrong, the business fallout can be costly.
Recent cases have made this crystal clear. For example, Air Canada’s chatbot misled a traveller about a discount, leading to a costly legal dispute that highlighted the airline’s accountability.
Meanwhile, after an update, DPD’s AI-powered support bot went off the rails, swearing at a customer and criticising its own services. The incident went viral, attracting unwanted attention and damaging the company’s reputation.
These examples and many others show how AI can easily transform from a helpful tool into a public relations nightmare. When AI tools make mistakes, businesses pay the price, both in terms of money and lost trust.
RAG tackles these challenges head-on. By connecting AI systems to external knowledge sources, including proprietary data, RAG allows them to access accurate, up-to-date information in real-time.
A recent study by 451 Research in collaboration with Salesforce found that 87% of enterprise leaders view RAG as a viable way to prevent hallucinations and boost trust in AI systems.
James Curtis, Senior Research Analyst at 451 Research, reinforces this idea: “RAG deployments can help quell enterprise concerns about trust, bias, and cost.”
Curtis highlights that RAG doesn’t just provide better outcomes; it simplifies AI adoption for businesses, making it easier to manage and scale.
Key benefits that make RAG an appealing option for organisations to include:
- Improved Accuracy: RAG dramatically reduces hallucinations by grounding responses in current, verified data sources, making it particularly valuable for industries that rely on up-to-date knowledge.
- Cost Efficiency: Instead of spending resources retraining AI models, RAG allows businesses to update knowledge bases easily and more frequently, reducing operational costs and the environmental impact of energy-intensive training processes.
- Security and Compliance: With RAG, sensitive or proprietary data is stored separately in a controlled vector database, helping businesses maintain compliance with security standards, which is particularly important in sectors like finance and healthcare.
The business case for RAG is growing stronger as more companies recognise the need for AI systems that can access specialised knowledge, surpassing the limitations of generalist models.
Driving Success with Domain-Specific AI
With domain-specific AI, you take control of the data that powers your systems.
Whether you’re building a knowledge base from scratch or enhancing existing models, this approach optimises AI to your specific needs while enhancing performance.
Through RAG (Retrieval Augmented Generation), your AI can access real-time, specialised knowledge, ensuring precise and reliable outcomes by tapping into the most relevant data.
By capturing your organisation’s expertise – whether in healthcare, finance, agriculture, or any other field – you can scale this knowledge across your operations. This means smarter, more accurate decisions driven by data that reflects your unique challenges and goals.
Aya Data can help you build or augment your AI models with the high-quality, domain-specific data that sets your business apart.
Ready to unlock the full potential of your AI? Contact us today to start creating your own domain-specific advantage.
Also Asked Headlines
What is the difference between GPT and RAG?
GPT (Generative Pre-trained Transformer) is an AI model that generates text based on pre trained data. RAG (Retrieval-Augmented Generation) improves GPT by retrieving relevant external information before generating responses, making outputs more accurate and reliable.
What is the future outlook for RAG in AI development?
As the demand for accurate and context aware AI systems grows, RAG is expected to play a pivotal role in enhancing the reliability and applicability of AI-generated content across various industries.
In which applications is RAG particularly useful?
RAG is beneficial in applications requiring accurate and contextually relevant information, such as question-answering systems, conversational agents, and domains where up-to-date knowledge is crucial.