Drones or UAVs (Unmanned Aerial Vehicles) are propelling a revolution in farming and agriculture.
In 2019, the FAO published E-Agriculture in Action: Drones for Agriculture, a detailed guide and roadmap of how drones are being used in agriculture, and the best practices for the future. The agricultural drone market is growing and is expected to surpass $4.8 billion by 2024.
The uses of drones in agriculture are still expanding. As well as mapping terrain and providing high-resolution birdseye images for both manual and automatic assessment by farmers and agriculturalists, drones are being used to phenotype crops, emit fertilizers and even sew seeds automatically.
Companies like DroneSeed are using drones equipped with seed cannons to replant seeds in areas ravaged by forest fire six times faster than if they were being planted by hand.
This article will explore how drones are being used to enhance crop yields with the assistance of computer vision and machine learning.
NDVI and Crop Yield
Modern agricultural drones can be equipped with high-definition cameras and hyperspectral, multispectral, and thermal sensors.
The first benefit of crop imaging is providing detailed images and video for manual analysis.
This provides a previously rare opportunity for agriculturalists to survey their crops from above without the use of helicopters which are vastly more expensive and non-sustainable compared to electric-powered lightweight UAVs.
Using drone footage, spectroscopic analysis then allows agriculturists to analyze crop health and estimate yield. The key measure here is Normalized Difference Vegetation Index (NDVI) which is already employed in satellite imagery to measure the health of large bodies of vegetation, e.g., rainforests.
NDVI is calculated by the differences in visible and near-infrared light reflected and absorbed by vegetation.
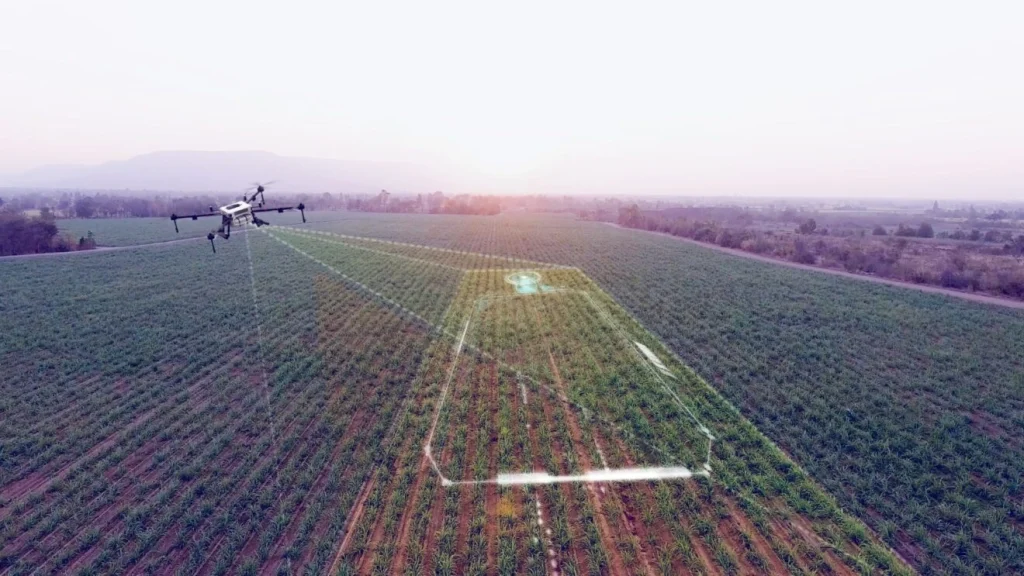
- Healthy vegetation absorbs the most visible light but reflects a lot of near-infrared light.
- Unhealthy vegetation reflects more visible light and also reflects less infrared light than healthy vegetation.
Studies have demonstrated that NDVI alone can estimate crop yields with a 7% error margin.
Whilst NDVI enabled by infrared imaging provides generally excellent results for estimating crop yield, computer vision takes it a step further.
Computer Vision and Crop Yield
NDVI provides a rough measure of vegetation coverage but fails to provide a deeper analysis of crop health.
Computer vision algorithms trained with customizable data labels can analyze crop health and many other phenotypes in-depth. Crop phenotyping is time-consuming and laborious, and phenotyping crops across a large and diverse geographical area is challenging indeed.
Drones equipped with high-quality sensors and cameras are scaling crop phenotyping up dramatically, allowing farmers to analyze their crops across huge and diverse geographic areas.
Drone-enabled high-throughput plant phenotyping (HTPP) represents a new generation in scalable crop phenotyping.
One study, published in The Journal of Horticulture Research used high-res NDVI images to label 100,000 lettuce signals which were used to train a supervised machine learning model.
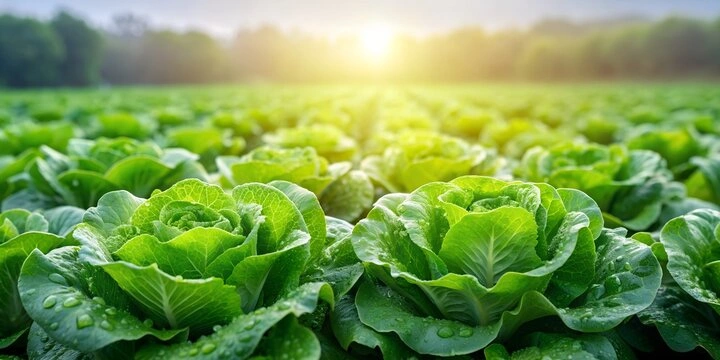
The model categorized and scored the number of individual lettuces with 98% accuracy. The model could discern the coverage of crops and estimate the size of individual lettuces.
This provides growers with clues to how well lettuces are growing in specific spots within a potentially massive area, allowing them to target problem spots with fertilizer or irrigation.
Other projects have used non-NDVI standard RGB camera images to train machine learning algorithms using bounding box, semantic segmentation, image annotation, and polygon annotation.
The potential of these techniques is limited only by the detail supplied by the images – machine learning algorithms are ideally equipped for utilizing the huge quantities of data that high-res images can provide, so long as the algorithms are well-trained and accurately validated.
Current and future projects hope to distinguish between different crops in multi-crop fields, identify disease symptoms, autoscreen for mutations, and detect small changes in plant growth.
The Benefits of Computer Vision and Crop Phenotyping
Drones are electrically powered, lightweight, and non-invasive. Once farmers receive training on how to use them, they have a long life expectancy and are more sustainable and efficient than manual sampling and analysis.
Computer vision enables farmers to interpret and analyze their crops at scale on a near pixel-by-pixel basis.
The benefits of combining drone footage with computer vision include:
- Seeking out areas of a field that are less productive than in previous years, highlighting them as a sight for increased fertilization, irrigation, or other interventions.
- Helping growers prioritize use of pesticides and fertilizer to not waste products on areas of the crop that are meeting or exceeding their growth targets and health.
- Discovering disease and screening for mutations. Computer vision algorithms can be trained using data labels for particular disease symptoms.
- Feeding AI-interpreted data into automated systems for automatic harvesting, weeding, feeding, fertilizing, etc.
- Sharing data with other farmers to help classify newly developing and ongoing problems with crops in a particular local area or region.
- Distinguishing individual crop phenotypes in multi-crop agriculture.
Summary: How Drones and Computer Vision are Used to Enhance Crop Yield
NDVI images obtained from drones provide a fairly accurate means to measure crop yield and growth characteristics at scale.
However, NDVI has given way to a new generation of computer vision techniques that utilize drone images to classify numerous characteristics associated with everything from plant size and development to disease, mutations, or even the presence of weeds.
In time, drone UAV devices will prove to be one of farming’s deadliest weapons in sustainably improving crop yields, reducing wastage, and driving new levels of agricultural efficiency.
Ready to boost your crop yield with the power of our reliable solutions? Speak to an expert today.
Discover how a bespoke solution was developed using drones and AI to increase efficiency and reduce risk in reforestation projects in our case study.